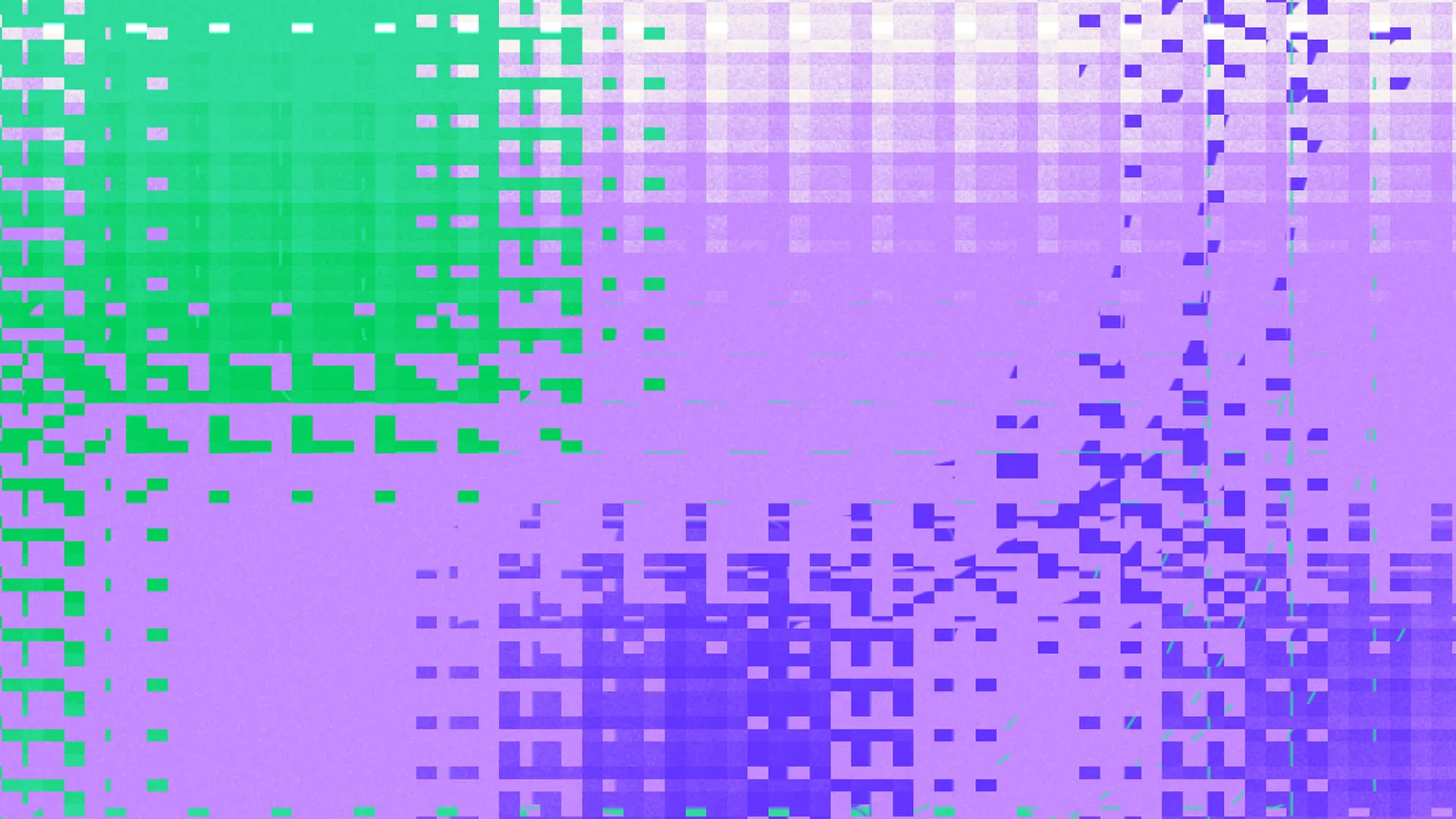
AI/ML
How Netflix, Lyft, and Yahoo use Contextual Bandits for Personalization
Inspiration from some of the world's biggest tech orgs
Learn more
As part of Eppo’s Humans of Experimentation conversation series, we sat down with Azadeh Moghtaderi, the Vice President of Data at Coursera.
In past roles, Azadeh led a wide range of Data functions (Data Science and Machine Learning) at Ancestry and eBay. Azadeh has spent the bulk of her career establishing ML/AI strategies and leading Machine Learning and Machine Learning Engineering teams to improve product and business metrics.
In this conversation, Azadeh explains:
What is a data team responsible for, and not responsible for?
What a data team is responsible for is shaping and influencing business decisions through ML/AI modeling and data-driven insights. We see ourselves as strategic partners, aligning our objectives with the wider business goals, helping stakeholders in asking the right questions, and validating our insights through methodologies like A/B testing.
It's crucial, however, to navigate the common pitfall of a data team becoming merely data wranglers or service providers. While we generate and validate insights and models, we also collaborate with various departments for the effective application of these insights. This partnership approach fosters a perception of the data team as a valuable partner and equal.
You’ve been working with AI since before it was the biggest topic in tech. How did it support business objectives at Ancestry and Coursera?
In my previous roles, AI has been a powerhouse supporting a range of business objectives.
Within the content space, generative AI was essential - we used computer vision and natural language processing for effective processing and extraction of information from images and text, significantly enriching our content and publishing efficiency.
AI has also been instrumental in enhancing product experiences. My long-standing investment in improving discovery mechanisms, particularly search and recommendation algorithms, has resulted in heightened user engagement and satisfaction by offering more personalized experiences.
For customer acquisition and retention, machine learning and data science were key to understanding customer behavior triggers and drivers. AI enabled more precise marketing strategies like personalization, propensity modeling, and targeted marketing, leading to increased customer retention and satisfaction.
Across all these areas, AI served as more than just a tool; it was a transformative force that consistently enabled us to address key business challenges.
Does Machine Learning require AB experimentation to be effective?
Machine learning and A/B experimentation go hand in hand when it comes to effective implementation. Simply put, it's challenging to validate the business impact of a machine learning model without proper experimentation.
When developing a machine learning model, the objective is to optimize a certain outcome and outperform the existing baseline. You can certainly validate your model offline, but the real test of its efficacy comes when it's exposed to customers in an A/B test.
A/B testing enables you to objectively evaluate which version of the model performs better under established criteria, thereby determining your new baseline. So, whether you're comparing two versions of a machine learning model or a machine learning model against a rule-based one, the goal is always to improve upon certain objectives. Ensuring these objectives are accurately measured online through customers' experiences is crucial.
So you can form an educated guess via machine learning, but the real world always surprises you.
Absolutely. The real world often throws curveballs that can only be addressed through testing. Search-ranking provides a prime example. We commonly use offline metrics like NDCG, or Normalized Discounted Cumulative Gain, which measures the quality of your ranking model.
While we know there's a positive correlation between this offline metric and search success, the specific impact isn't always clear. The only way to truly gauge the magnitude and significance of it in terms of business impact is to run an A/B test.
What's the connection between generative AI and experimentation, and how has the work of data teams changed? Some leaders have suggested to me that ChatGPT’s feedback loop is a form of experimentation, because when you tell ChatGPT that its answer is unsatisfying, you’re training the model to do better. But that isn’t the same thing as AB experimentation.
Generative AI and experimentation certainly intersect. Just like any other AI or machine learning model, you can run A/B tests on generative AI outputs.
Here are a couple of examples. If your generative AI model is a large language model, it could generate two versions of a marketing email subject line. These versions can be used in an A/B test to determine which leads to a higher open rate. Similarly, generative AI imaging could create different iterations of a website for testing. Large language models like ChatGPT allow for rapid adjustments in 'prompt engineering' without requiring a complete model retraining. This efficiency is a game-changer: rather than spending time retraining models, you can adjust your prompts and run tests quickly.
Generative AI has thus introduced significant efficiencies in testing and learning. It allows for faster, more frequent input generation, paving the way for more robust and agile testing.
Can generative AI also help experimentation quality itself?
Certainly, generative AI can enhance the quality of A/B testing. For instance, in a multi-armed bandit testing framework, generative AI can help estimate the reward distribution across different test options or 'arms' more efficiently. Instead of equally distributing probabilities across all exploration cases, generative AI can use existing data to learn this distribution, streamlining your A/B test's exploration phase.
This technique also aids in balancing exploration (trying out new options) and exploitation (focusing on the best-performing option). By generating new versions of what you want to test rapidly, generative AI can speed up the exploration process. Meanwhile, the exploitation layer can concentrate on optimizing the best-performing option.
Essentially, generative AI helps your tests dynamically move towards the better outcome while the test is running, reducing the time for exploration, and enabling faster convergence on the optimal solution.
Today’s Generative AI products are largely built off the same handful of foundation models, so it seems like differentiation and defensibility is only going to become more important.
As generative AI products continue to be built on the same foundational models, differentiation and defensibility indeed become more important. Customer demand remains the core problem, and the only way to ensure alignment with it is through rigorous experimentation and rapid iteration.
Generative AI comes into play here as a tool that significantly accelerates this process. Instead of spending months building something to test, we can now quickly iterate and bring it to the testing phase. This doesn't mean the need for A/B testing has diminished. In fact, it's more crucial than ever.
You’ve spoken about the importance of not introducing bias into your AI. How does experimentation help with that?
In AI, there's a mix of rule-based and algorithmic approaches. While human logic forms the foundation of rule-based systems, these can introduce bias because they're inherently shaped by our experiences and perspectives.
When these biased rules form the basis for machine learning models, the resulting algorithms may perpetuate these biases. Unless we're intentional about addressing this, our models will only learn from the data we feed them, bias included.
This is where experimentation comes in. Through an experimentation-based evaluation, we can detect and measure these biases. We can then adjust our models or the data they're learning from to reduce the impact of the bias. By doing so, we ensure our AI systems are not just replicating our biases, but are learning and evolving in a more balanced and fair way.
What’s the most exciting thing about the data space right now?
What truly excites me about the data space right now is the potential of generative AI to transform the way we innovate. As we integrate these tools into our everyday tasks, we're discovering that we can work faster, become more efficient, and focus our energies on solving more interesting and complex problems. For instance, I use ChatGPT as a thought partner every day. It helps me articulate my thoughts more effectively, freeing me up to tackle more significant issues.
While generative AI presents a fascinating data challenge, my primary interest lies not in its impact on data teams but on the world at large. The possibilities are truly boundless.
To learn more about why AB experiment infrastructure is essential to AI, read Eppo's AI manifesto.