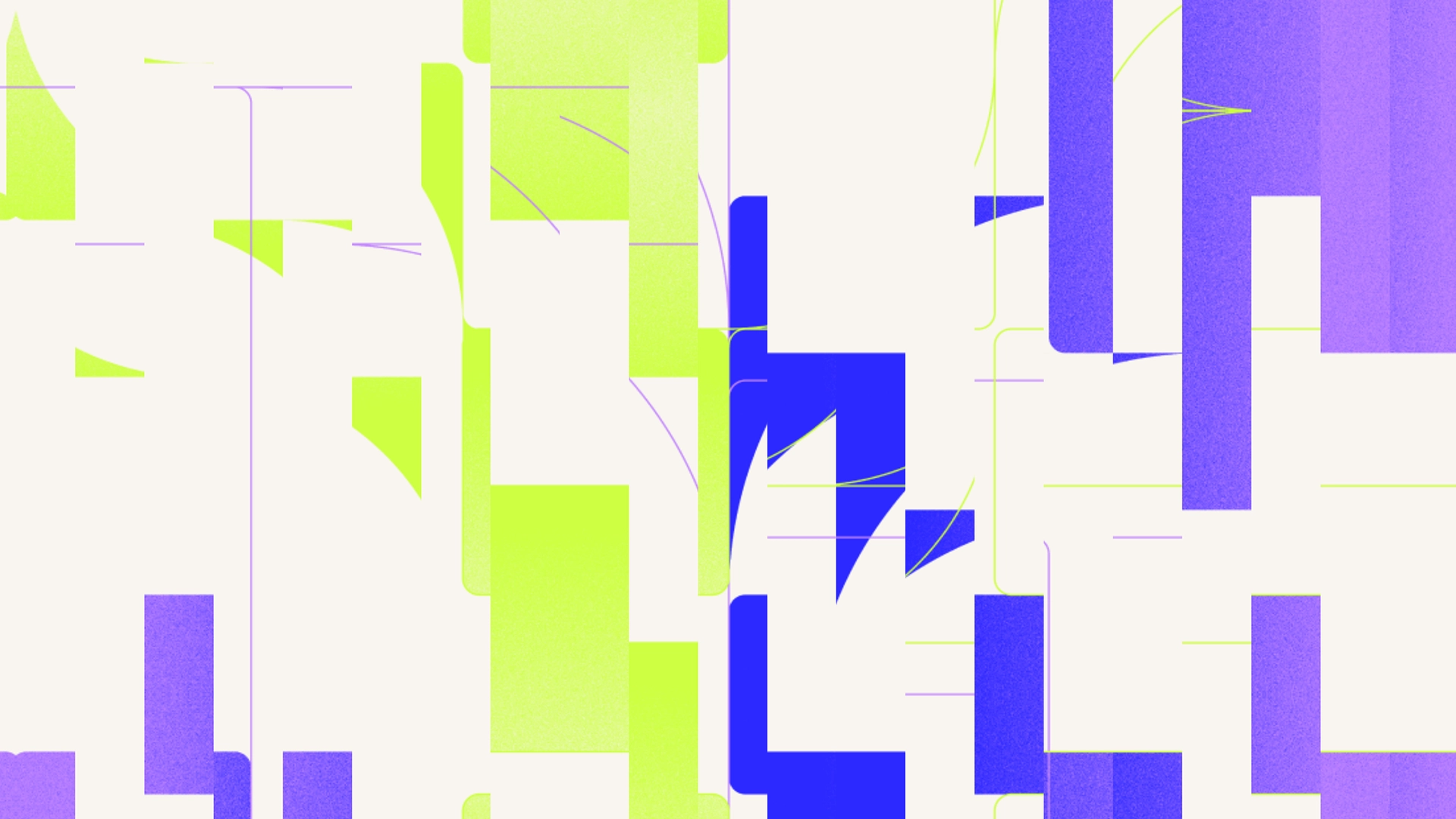
Strategy
Mastering Experimentation Methods: From Design to Analysis
Learn more
When optimizing products, immediate feedback metrics like clicks, signups, or purchases offer quick insights for testing and iteration. Yet, the most impactful business metrics often take much longer to surface, such as:
These long-term metrics pose a distinct challenge. Relying on them delays optimization efforts while focusing solely on short-term signals risks prioritizing quick wins at the expense of sustained business success. This creates a core tension between speed and thoughtful, long-term outcomes.
Optimization techniques like A/B testing and multi-armed bandit algorithms both excel with instantaneous feedback loops, but serve complementary purposes. A/B testing provides rigorous statistical validation of specific hypotheses, while bandits enable dynamic optimization through rapid exploration and exploitation. Both approaches are particularly valuable for products with short decision cycles, as their results can drive rapid iteration and can be effectively combined to balance statistical confidence with adaptive learning.
However, problems arise when immediate metrics fall short in representing long-term success, such as in these scenarios:
These factors make optimizing for the long run both challenging but no less essential, demanding a thoughtful balance between patience and agility.
Proxy or surrogate metrics act as early indicators that align closely with later, more valuable outcomes. Essentially, they serve as substitutes for outcomes that are either not yet observable or inaccessible due to data limitations.
The process involves pinpointing metrics that appear relatively early in the customer journey and consistently predict downstream outcomes. If a software subscription offers a three day free trial, the point at which users add a credit card – showing value and an “aha” moment – might be the metric to focus on. For instance, if data shows that 80% of users who put in payment info during the first week ultimately become long-term customers, then driving seven-day “add payment” becomes a practical proxy for optimizing marketing efforts.
While surrogate metrics can be powerful, they come with key challenges:
By using surrogate metrics thoughtfully, businesses can bridge the gap between short-term visibility and long-term value, but the approach demands ongoing validation and adaptation.
Eppo offers Contextual Bandits, a powerful implementation of “multi-armed bandit” algorithms that help test choices and then scales the winners automatically. Bandits ingest short-term rapid metrics so they can rapidly adapt to changing circumstances, which creates the need to monitor longer-term metrics for business impact alongside it. Nested optimization merges the adaptability of bandit algorithms with the methodological rigor of A/B testing. This approach layers short-term optimization (via bandits) within long-term experimentation (via A/B tests), creating a framework that addresses both immediate feedback and long-term outcomes.
An effective implementation might look like this:
Predictive modeling upgrades surrogate metrics to incorporate multiple features or attributes into making a more accurate prediction of user behavior up front. These models, trained on historical data, allow organizations to forecast long-term outcomes for current experiments, bridging the gap between immediate insights and extended timelines without relying on delayed metrics.
You may choose to train a model that predicts outcomes like lifetime value (LTV) or churn probability within the first few days or weeks of a user’s engagement with your service.
As opposed to simply using a surrogate metric, modeling can capture some important nuances, like:
These types of models are an important tool for many different kinds of digital measurement, not just for metric optimization on-site or in-app but also in marketing campaign optimization. Meta recently open sourced a new tool, LTVision, that aims to make it easier for advertisers to incorporate their first-party data into advertising optimization.
Open-source tools like Meta’s LTVision exemplify the practical application of predictive modeling for long-term value.
When analyzing experiments involving long-lead metrics, teams face an important methodological question. Should they:
The answer depends on specific circumstances, including:
Both approaches have merit in different situations, and experimentation platforms like Eppo support flexibility in this regard.
Eppo’s experimentation platform is designed to support all three strategies for tackling long-lead metric optimization, offering adaptability to suit varying business needs and data availability.
Eppo enables teams to define metrics at the entity level (e.g., a user), allowing flexibility in how different aspects of the business are measured. This capability supports implementation of the following approaches:
When conducting analysis, Eppo offers flexibility in cohort inclusion methods, empowering teams to choose between two approaches:
Organizations addressing challenges related to long-lead metrics should weigh several factors to determine the most appropriate optimization method:
Often, the best solution combines multiple strategies, tailoring them to the organization’s unique challenges. By thoughtfully addressing the complexities of long-lead metrics, teams can preserve the fast pace of experimentation while keeping long-term business goals firmly in sight.
Effectively optimizing for long-lead metrics involves striking a balance between the need for quick experimentation and the focus on long-term business impact. Organizations can address this challenge by leveraging proxy metrics, implementing nested optimization strategies, using predictive models, or combining these methods to suit their needs.
The most suitable approach will depend on your organization’s unique business circumstances, data maturity, and technical expertise. Whichever path you take, maintaining a dual focus on both early indicators and long-term outcomes will ensure your optimization efforts contribute to meaningful and sustainable business growth.