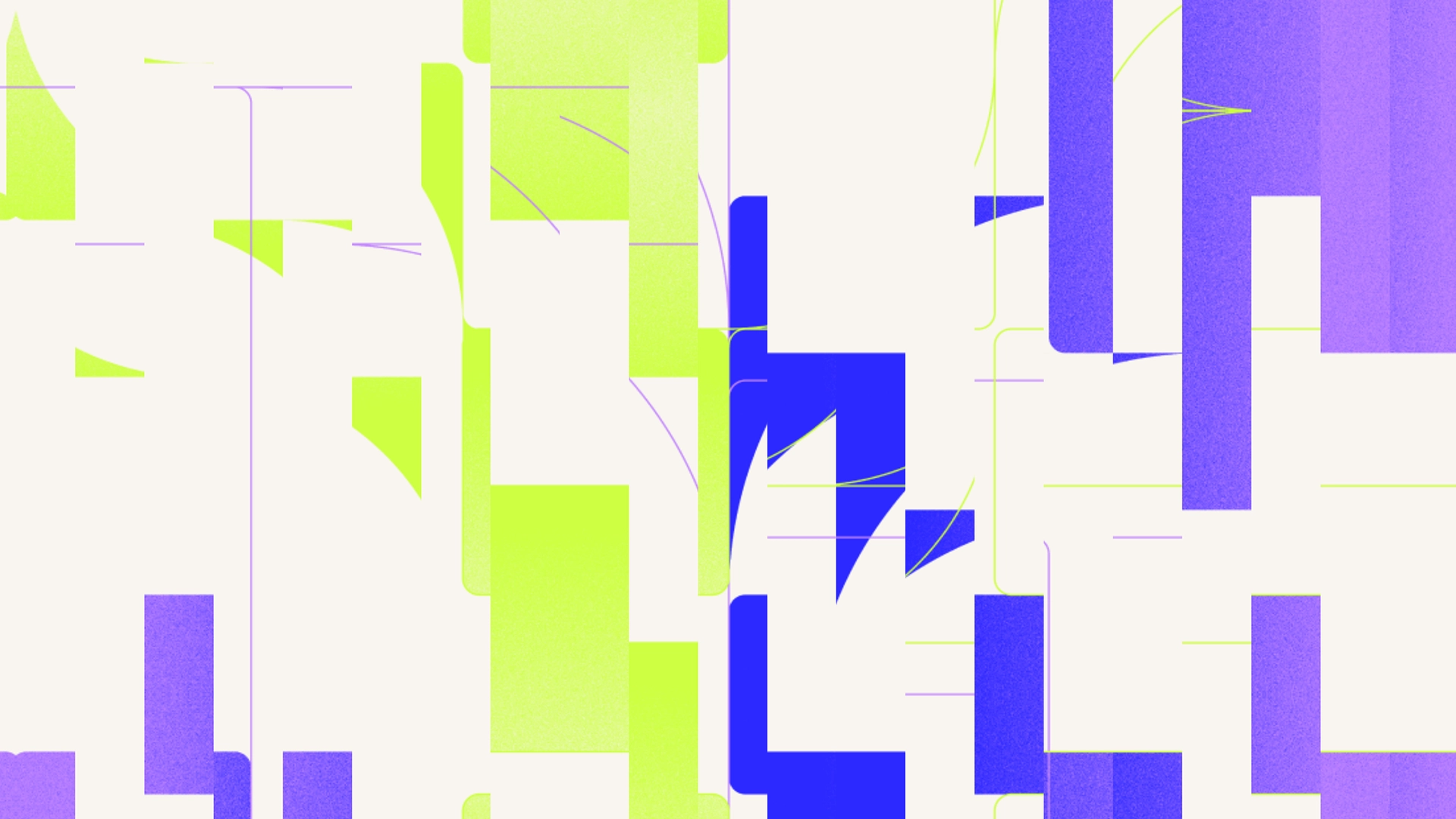
Strategy
Mastering Experimentation Methods: From Design to Analysis
Learn more
If you've worked in experimentation, you're probably familiar with the rush of seeing a successful A/B test. A key metric climbs in the right direction, and a feature gets declared a "win." Over time, as more experiments succeed, it’s tempting to add up all those positive results. After all, the numbers suggest that these “lifts” are adding up to massive overall gains for the product or business.
But then comes the sobering reality check. The product’s overall metrics don’t reflect the level of improvement the experiment results implied. What went wrong? It turns out that simply summing up experiment wins often paints an overly optimistic picture of true impact. This is where experienced experimentation teams use a strategy called holdouts to get a clearer view of cumulative performance.
If you’re not familiar with the term, a holdout (or global control group) is a subset of users or traffic that doesn’t receive any of the new features being tested. By comparing the overall performance of the holdout group with the rest of the user base that experiences all the changes, teams get an accurate measure of how much all the experiments combined have actually moved the needle. And more often than not, the gains revealed by the holdouts are far less dramatic than the naive summation of individual “wins.”
But why is there such a gap between perception and reality? Fortunately, there are steps you can take to avoid falling into these pitfalls and ensure your experiments deliver real, measurable results for your product or business. Let’s dive in!
It’s not just bad luck that the cumulative effect of individual experiments often fails to match expectations. Several key factors contribute to this overestimation:
The “winner’s curse” is a pervasive issue that subtly skews the way we interpret experiment results. Borrowed from auction theory, it describes the phenomenon where the winner tends to overpay due to relying on overly optimistic estimates. The same dynamic occurs in A/B testing.
When we only launch experiments that achieve statistically significant results, those we call "wins", we’re knowingly cherry-picking the most positive outcomes. This means some of the observed lifts reflect random noise rather than true underlying effects. Features that appear promising in tests may have simply benefited from a statistical fluke, especially when many tests have been run or when tests are underpowered.
The winner's curse issue compounds when statistical power is low. For example, in small-sample experiments or tests with marginal effect sizes, random variability is more likely to play a role in hitting the significance threshold. Decisions made based on "wins" are, therefore, prone to overestimating their real-world value.
Experiments are typically conducted in isolation, under the implicit assumption that the effect of one feature is independent of another. This assumption rarely holds true in complex products where new features often overlap in audience, goals, or mechanics. These interactions can lead to inflated cumulative estimates.
Common types of interaction effects include:
Most online experiments are evaluated on relatively short timeframes. Short-term lifts are quick to measure and easy to align with product timelines, but they may not represent sustained user behavior changes.
A common example of this mismatch is the novelty effect, where a new feature grabs attention simply because it’s new. Over time, as users adjust, its impact may fade. If multiple tests capitalize on this transient novelty and their results are summed, the total figure exaggerates the enduring value.
Compounding this issue is long-term decay. If experiment results are snapshots in time, summing them assumes all those short-term lifts will persist indefinitely and simultaneously. This simply isn’t the reality for most products.
Experiment design and analysis practices can also inflate individual results. While well-intentioned, certain biases and behaviors compound the issue:
This is where Eppo stands out. Unlike manual or ad hoc approaches, Eppo standardizes analysis and enforces rigorous methodologies that mitigate these common pitfalls. By embedding best practices into the experimentation process, Eppo ensures teams don't fall prey to selective reporting, stopping bias, or cherry-picking metrics. Instead, it delivers a clear and unbiased view of experimental outcomes, providing confidence in the results and their real-world applicability.
How can teams counteract these pitfalls? Several approaches have emerged to measure true impact more accurately and minimize biases.
A holdout is a subset of users who are excluded from receiving any new features over a given timeframe. This group serves as a baseline to compare against the rest of the user population, providing a direct measure of cumulative impact.
Leading tech companies like Facebook and Microsoft use global holdouts extensively. For example, Facebook rotates its holdout groups every six months and measures the aggregate product impact of all new features during that half-year period. Microsoft employs “holdout flights” to validate individual experiments and the overall impact of product changes.
Beyond the larger tech companies, holdouts are less rare. For many companies, the downsides outweigh the benefits. In particular, it is difficult to power holdouts unless a company has millions of users. Furthermore, running holdouts adds a drag on engineering productivity because older versions of the application need to be supported.
Luckily, there is a great alternative for those who are not able or willing to use holdouts:, Bayesian methods offer a powerful statistical alternative. By adjusting experiment results based on uncertainty, Bayesian shrinkage provides a corrected, less exaggerated estimate of impact.
The logic is intuitive; an observed effect that comes with high uncertainty likely needs to be pulled toward the average. Sites like Etsy have adopted similar Bayesian approaches to reframe how teams interpret and report experiment results. Note that even frequentists should appreciate this result, due to connections with “James-Stein” estimation.
There are also simpler, non-holdout methods to reduce overestimation:
To build a robust experimentation program, it’s essential to instill the right habits across teams.
Here’s what works in practice:
The temptation to sum up experiment wins is understandable—it provides a seemingly straightforward way to quantify success. However, overestimation not only risks underserving both organizations and users but also undermines the very practice of experimentation by promoting flawed methodologies and eroding trust in data-driven decision-making.
Leveraging global holdouts, Bayesian adjustment, and enhanced analysis techniques allow teams to bridge the gap between perceived and true impact. By doing so, experimentation becomes not just a tool for delivering statistical wins but a disciplined approach to driving meaningful, validated outcomes. Remember, it’s not just about achieving numbers on a dashboard; it’s about delivering value you can trust.
Curious about how to measure true impact without the pitfalls of overestimation? Eppo's experimentation platform is designed to help you uncover accurate results, reduce biases, and make data-driven decisions with confidence. With advanced tools like holdout analyses and Bayesian correction baked in, Eppo empowers teams to turn A/B testing into a reliable growth engine. Take control of your experimentation strategy and learn more about Eppo today.