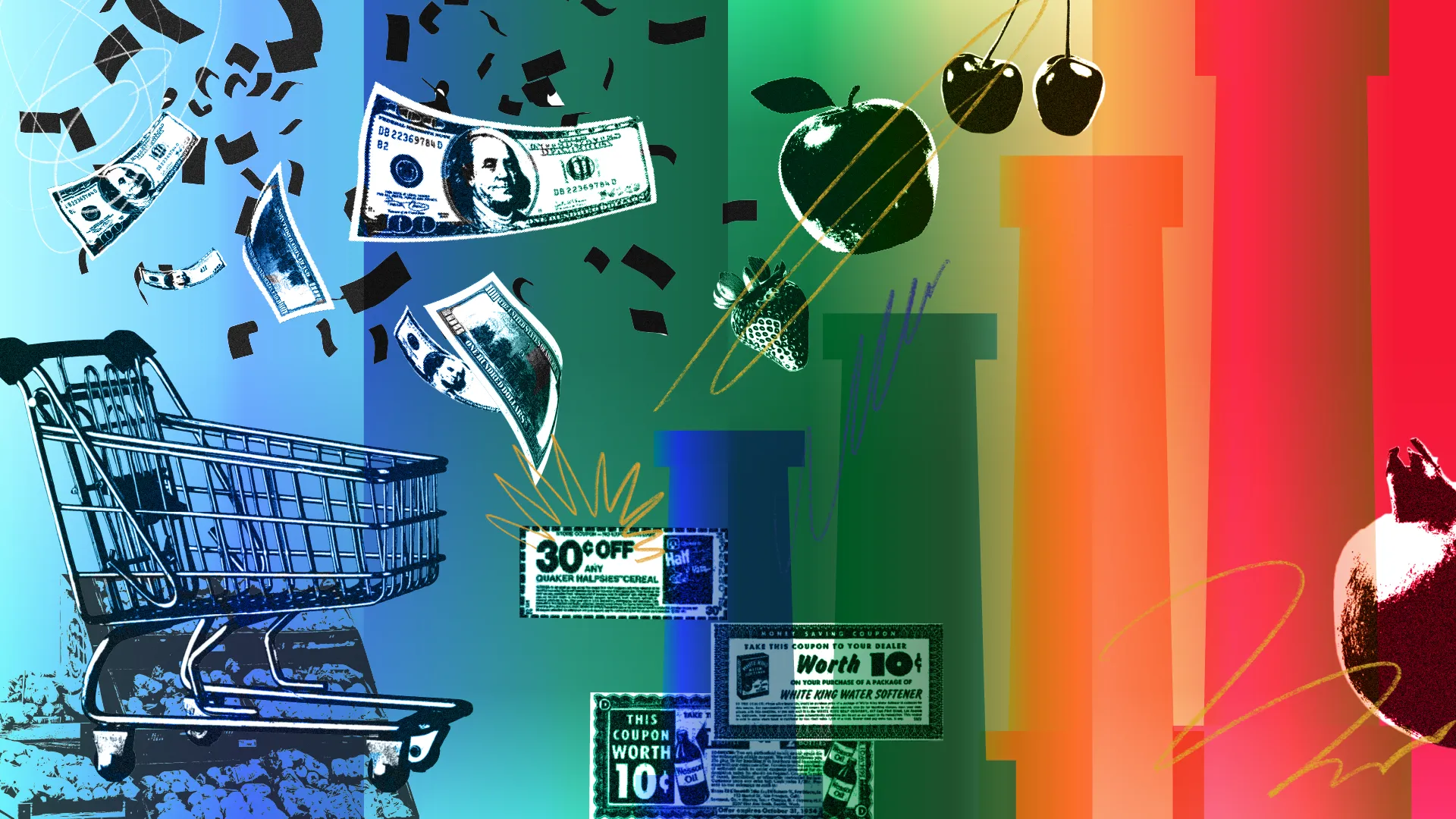
A/B Testing
Optimizing for Real Business Impact: A Strategic Framework for Ecommerce Experimentation
Learn more
Businesses learn faster when everyone is experimenting. But getting experimentation adoption across engineers, marketers, and PMs with diverse skillsets can be a slog. An excited engineer quickly realizes they have to navigate data warehouses, statistical methodology, metric tradeoffs, and potentially a bureaucracy of “experiment committees”, all just to test an idea they think might work.
The implementers of ideas - the engineers coding features and marketers spinning up campaigns - deserve a better arena to test and prove these ideas, one that requires no additional skilling of stats or metrics. Today, Eppo sets a new standard for self-serve experimentation with two new features: Protocols and Property Analysis.
Protocols create streamlined, vetted paths for experimenters to run tests without having to make specialized decisions. Companies can create protocols for all common buckets of tests, such as “search ranking experiments” or “growth experiments”. Here’s an example set of decision points that’d normally require tribal knowledge, but that this company instead preset with an Eppo Protocol:
activations
as primary metric, also check signups
and subscription upgrades
along with guardrail metrics. Decide on experiment success according to a decision matrix.activations
are up and signups
are down, ok to launch.activations
is up but subscription upgrades
are down, don’t launch.Instead of having to know all of these details, our junior growth engineer using Eppo Protocols only has to know the details in her purview: a.) how to build the products and b.) that her experiment is a “growth experiment”. Other experimenters can use protocols built for their use case, such as a “pricing experiment” that requires stricter rollout approvals, or an “AI model evaluation” that includes percentile latency metrics and using a particular mutually exclusive layer.
Eppo Protocols can be used as experiment templates, easing experiment setup with prefilled options that can be readily changed. But larger experiment orgs can choose to use Protocols as a governance regime, up-leveling rigor across teams at scale. When used for governance, running experiments with Protocols requires no consultation or oversight. When necessary, deviating from set Protocols will require a simple approval from designated users. Removing this friction accelerates the number of experiments that can be run, with a set quality bar.
Property Analysis also streamlines experiment workflows, but where protocols focuses on setup and decisioning, property analysis boosts learning velocity via automated deep dives. The experimentation process involves an analysis period where every stakeholder is hungry for answers for why an experiment succeeded or failed. This process usually relies on experts, typically in Data Science, to check the right dimensions and find those answers.
For example, a key metric like purchase conversion might be positive, but could it have unexamined downside on a key segment? Property Analysis allows for the junior engineer to proactively see that it had an outsized negative effect on new users, or users on iPhones, or Chinese users. The need for an expert is now removed, saving timing, surfacing insights, and resulting in better learnings.
When everyone can run experiments confidently, companies innovate faster. Protocols and Property Analysis remove the traditional barriers to experimentation adoption, transforming it from a specialized skill to a natural part of every team's workflow. Engineers can ship with confidence, marketers can optimize with precision, and product managers can validate hypotheses rapidly - all without needing to become statistical experts or navigate complex approval chains.
But this isn't just about making experimentation easier. It's about fundamentally changing how companies learn and grow. When teams can quickly test ideas and get rich insights automatically, they make better decisions, faster. The result? More innovations that work, fewer resources spent on initiatives that don't, and a culture where data-driven decisions become the norm, not the exception.
Ready to accelerate your company's experimentation culture? Schedule a demo today to see how Protocols and Property Analysis can transform your team's ability to learn and innovate at scale.