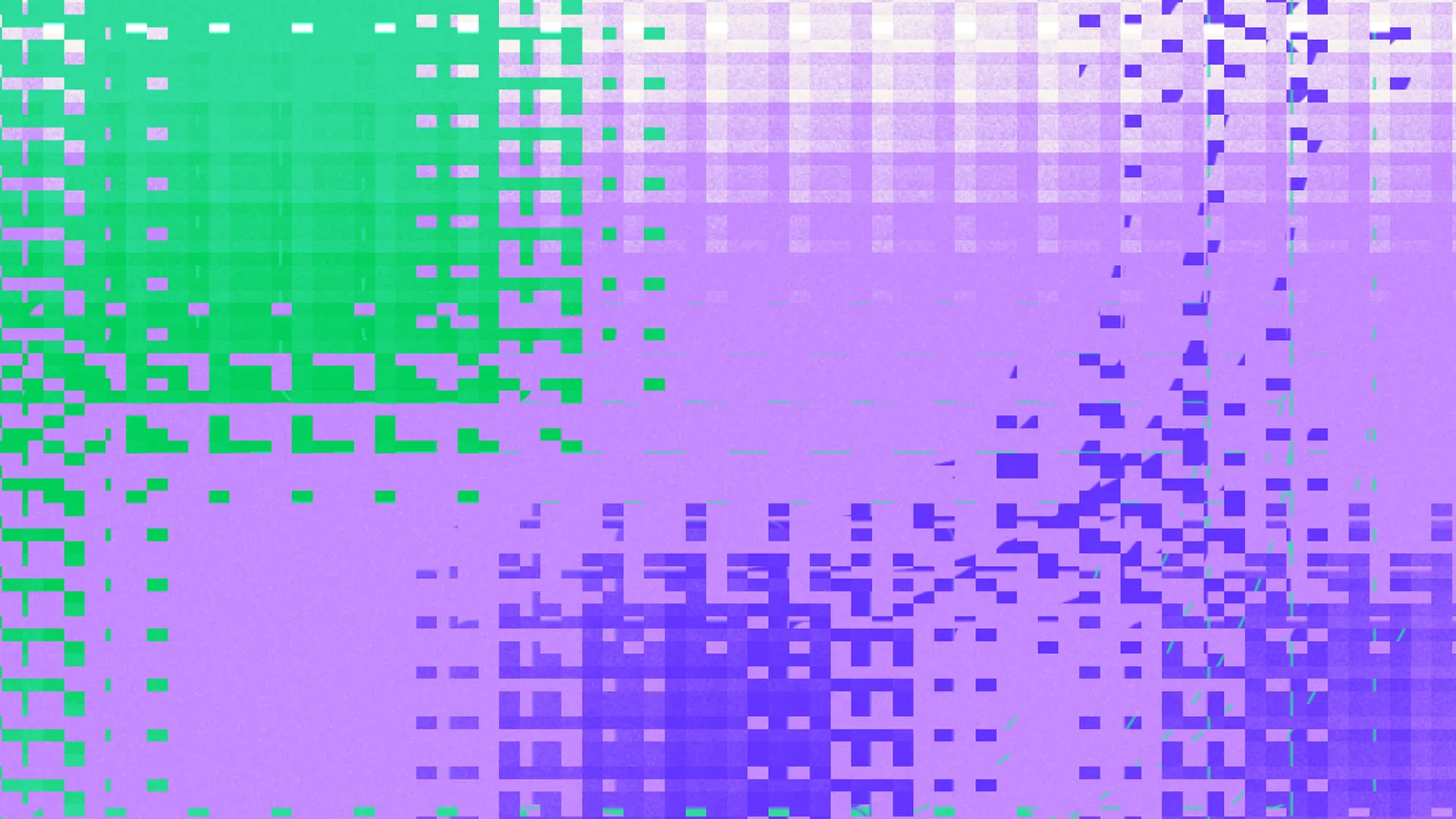
AI/ML
How Netflix, Lyft, and Yahoo use Contextual Bandits for Personalization
Inspiration from some of the world's biggest tech orgs
Learn more
Every company will soon be an AI company. There’s never been a lower bar for building AI. Where even simple AI deployments previously required an exclusive level of expertise - CS PhDs, data architects, Netflix alumni types - any company can now publish AI applications with the simplest of inputs: human sentence prompts.
But as the cost of deploying AI models goes to zero, companies are lost navigating the choices between models. Already, AI teams need to decide:
Every company can now deploy models, but winning companies can evaluate models. A cursory examination of top AI practices like Amazon, Netflix, Airbnb reveals the gold standard for evaluation: an obsession with streamlined evaluation via AB testing on business metrics.
Winning AI teams evaluate models the same way they evaluate the business. They use business metrics like revenue, subscriptions, and retention. But today’s MLOps tools instead lean on proxy metrics measured in offline simulations and historic datasets. Leadership teams must take a leap of faith that simulations will translate to real life.
These simulations rarely predict business impact. Decades of studies from Microsoft, Amazon, Google, and Airbnb reveal an inescapable reality that 80% of AI models will fail to move business metrics, despite glowing performances in simulation. There are plenty of reasons why these simulations don’t hold up, from data drift to mismatched historical periods or proxy metrics not translating to business metrics.
The gold standard for model evaluation is randomized control trials, measuring business metrics. Winning AI strategies connect traditional MLOps to the live production environment and core analytics infrastructure. Put simply, enterprise AI needs enterprise AB experimentation.
Legacy commercial experimentation technology is not ready for AI workloads. These offerings were built for marketing websites and click metrics, and not for AI model hosting services and business metrics. At a minimum, AI-ready experimentation infrastructure needs the following:
These gaps with legacy experimentation platforms have already been noted by today’s enterprises, who instead opted to create the same in-house tooling, over and over and over again. These in-house tools have become huge investments to maintain even as a down-market forces fiscal prudence.
Companies who are new to investing in AI need a faster, reliable way to achieve AI-grade AB experimentation workflows.
At Eppo, we believe that AI teams need to hear their customers. Generative AI gives companies superpowers, but doesn’t help these companies make sure the superpowers are used to customer benefit. An AI team that is divorced from business metrics will rapidly ship models, but will degrade the product without AB experiments. AB experimentation infrastructure is part of MLOps infrastructure.
Increased speed of development comes with cautionary tales of insufficient evaluation. It’s not difficult to find real-world stories of AI-powered failures, where trust in an “AI strategy” leads to a messy outcome without purpose built evaluation tools. Rapid development of models is only a superpower if the evaluation of those models is also rapid. AB testing is the key tool to do so, allowing accelerated innovation with AI, rather than becoming mired in a pool of mediocre, generated content.
Eppo is here to link AI investments to business outcomes, and make sure customers are part of every AI model decision.
This is the first part of Eppo's AI manifesto. To read Part II, "AI Enabled Creators Need AB Experiments," go here.