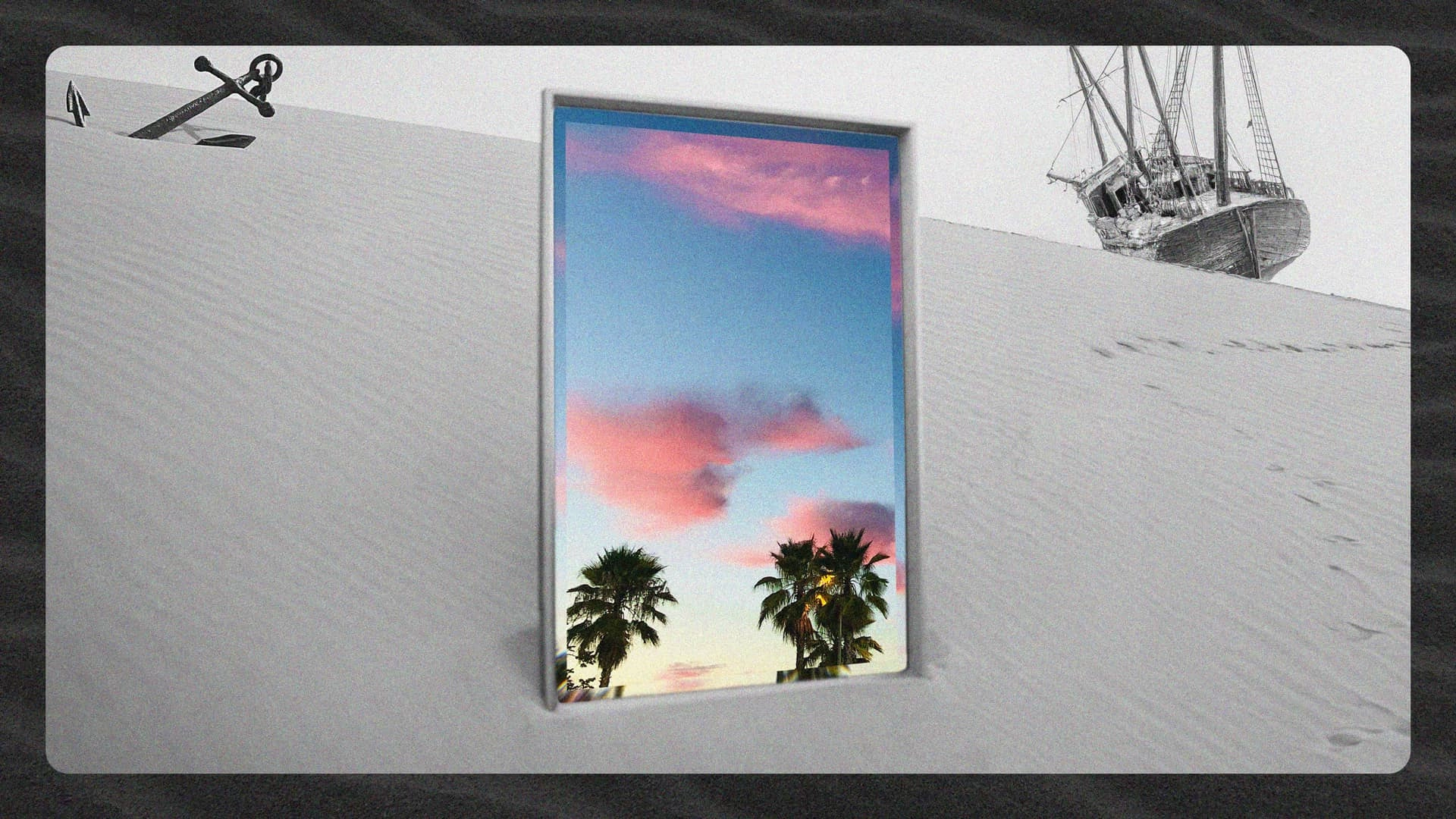
Statistics
The Illusion of Safety: How Non-Informative Priors Lead to False Confidence
Learn more
TL;DR:
Drawing accurate conclusions can be tricky in experimentation. Without a straightforward way to determine whether results are due to chance or a real effect, it's common to misinterpret random variability as meaningful, leading to false positives or inconsistent findings across experiments. You can combat this by setting a significance level, which provides a standard threshold for evaluating results and helps teams ensure that their conclusions are statistically reliable and actionable.
In this blog, you'll learn:
By the end, you'll know how to properly apply significance levels to your experiments and make smarter, data-driven decisions.
In hypothesis testing, we start with two competing statements:
A significance level (often denoted as α) is the threshold you set to decide if the results you see are likely due to chance or indicate an actual statistical change or impact. It is a benchmark for determining whether to reject the null hypothesis based on your data.
The concept of significance levels is widely used across modern experiment frameworks, but it wasn't always a standard. Ronald Fisher and Jerzy Neyman, two influential figures in statistical analysis, popularized it. Fisher introduced the use of significance levels to measure the strength of evidence against the null hypothesis. Neyman, alongside Egon Pearson, helped refine hypothesis testing by defining Type I and Type II errors. Their work laid the groundwork for the statistical methods we use today.
Once your null and alternative hypotheses are defined, you need a way to evaluate the strength of the evidence. This is where you'll need to employ your significance level (α) and p-value.
As we've already mentioned, the significance level is essentially a threshold you set before running the experiment that represents the maximum acceptable risk of rejecting the null hypothesis when it's true.
The p-value is the statistic you calculate after running the experiment. It tells you how likely the observed data is to occur under the null hypothesis. TheThe p-value gives you the strength of the evidence against the null hypothesis.
While it's always nice to have a cut-and-dry solution, keep in mind that, in hypothesis testing, we can see two types of errors that throw a wrench in the points we just made above:
Let's look at how choosing the right significance level can help you mitigate these errors.
The most commonly used significance level is 0.05, which means there's a 5% chance that you could mistakenly reject the null hypothesis when it's actually true, resulting in the type I error we mentioned above.
By setting your significance level at 0.05, you're saying, "I'm okay with a 5% risk that this result is just a fluke, not a true effect." In A/B testing, for example, if you see a bump in user engagement. However, your significance level is 0.05, there's still a 5% chance that bump could be random noise rather than something meaningful.
So, why does this matter? Well, the significance level you set shapes how much risk you're willing to take with your decisions. If you're in a high-stakes situation (think medical research or product safety), you may opt for a lower significance level like 0.01 to make sure you're not jumping to conclusions based on something that could be random.
However, in situations where speed and exploratory insights matter more than absolute certainty, like with smaller sample sizes in early-stage experiments, a higher significance level like 0.10 might be okay.
A common misconception is that a p-value less than 0.05 automatically means the alternative hypothesis is true. A p-value of less than 0.05 only means that the observed effect is unlikely to have occurred under the null hypothesis (i.e., no effect). It doesn't confirm that the alternative hypothesis is true. It simply suggests that the data provides enough evidence to reject the null hypothesis in favor of the alternative. Still, it doesn't guarantee the effect you observed is real or replicable.
For example, let's say you're testing a new feature in an app and get a p-value of 0.03. This means there's a 3% chance the observed improvement in user engagement could have happened due to random chance. But just because you've rejected the null hypothesis doesn't mean the feature works. The p-value only tells you that the data you've observed is unlikely to have happened by chance.
That's why it's so important to interpret the p-value in context, using additional analysis (like confidence intervals or effect size) to determine the practical significance of the experiment's result better.
A result might pass the statistical threshold (e.g., p-value < 0.05) but still have little real-world importance. For example, a new feature might increase revenue by 1%, which may be statistically significant, but the revenue boost might not justify the cost of developing or maintaining that feature. Consider whether the effect has real-world relevance before acting on statistically significant results.
The size of your sample plays a super important role in determining the statistical power of your test. Larger sample sizes reduce sampling error and make it easier to detect small effects, even if they're trivial. On the other hand, smaller samples can lead to a higher risk of Type II errors (false negatives), meaning you might fail to detect a real effect. In these cases, it's important to interpret results with a grain of salt because smaller samples may not provide the whole picture.
Instead of simply testing a hypothesis against a fixed threshold, Bayesian methods calculate the probability that a hypothesis is true given the data and prior information from historical data. Bayesian A/B testing can help make faster decisions with fewer samples, reducing the need for large sample sizes while still providing reliable insights.
The more tests you run, the higher the chance of finding a significant result by random chance. To account for this, techniques like the Bonferroni correction can adjust for multiple comparisons by making the significance level stricter. Another approach is controlling the False Discovery Rate (FDR), which helps manage the rate of false positives in large-scale testing. By controlling the FDR, you can ensure that the results you're acting on are more reliable, even when testing many hypotheses simultaneously.
Eppo is a powerful experimentation platform that simplifies the complexities of statistical analysis by automating key aspects of significance testing.
Eppo takes the complexity out of significance testing by automatically calculating p-values, confidence intervals, and effect sizes for you. This means you can trust that your statistical analysis is accurate without spending time on manual calculations. Eppo handles the heavy lifting, allowing you to focus on interpreting the results and making informed decisions.
Eppo delivers more reliable results by using tools like CUPED to reduce noise in the data. By minimizing variance, Eppo tightens confidence intervals and improves the precision of its results, even when working with smaller samples. This leads to more actionable insights and reduces the risk of misinterpreting the data.
Eppo's intuitive dashboards make it easy to visualize statistically significant results, confidence levels, and thresholds directly in the interface. With all your key metrics in one place, you can quickly assess your experiment's outcomes and make faster, data-driven decisions without getting bogged down by complex statistics. Ready to automate your experimentation? Request a demo today!