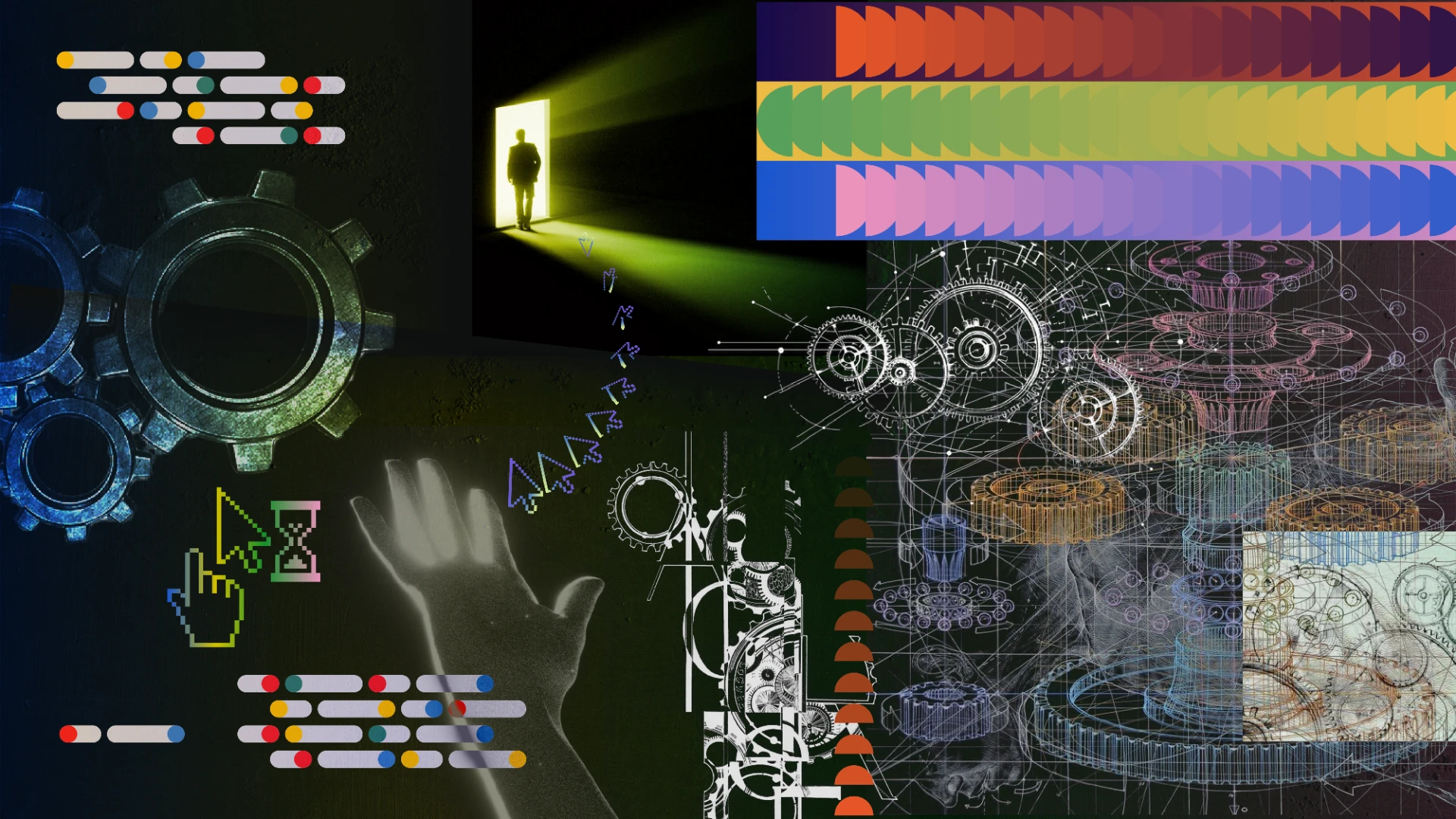
Engineering
What’s Wrong with Feature Flags?
Engineering and Growth teams aren't speaking the same language
Learn more
I started my career as a software engineer at Applied Predictive Technologies (APT), which sold multi-million dollar contracts for sophisticated AB testing software to Fortune 500 clients and was acquired by Mastercard in 2015 for $600 million. So I’ve been involved in AB testing since the beginning of my career.
A few years later I was VP of Engineering at Storyblocks where I helped build our online platform for running AB tests to scale our revenue from $10m to $30m+. Next at Foundry.ai as VP of Engineering I helped build a multi-armed bandit for media sites. Then a few years later I helped stand up AB testing as an architect at ID.me, a company valued at over $1.5B with $100m+ in ARR.
So I should have known a lot about AB testing, right? Wrong! I was surprised just how little I knew after I joined the AB testing platform company Eppo. Below is a synthesis of what I wish I’d known about AB testing when I started my career.
First things first, let’s define what AB testing is (also known as split testing). To quote Harvard Business Review, “A/B testing is a way to compare two versions of something to figure out which performs better.”
Here’s a visual representation of an AB test of a web page.
The fake “YourDelivery” company is testing to figure out which variant is going to lead to more food delivery orders. Whichever variant wins will be rolled out to the entire population of users.
For the rest of the article, I’ll be assuming we’re working on AB testing a web or mobile product.
Ok, so let’s define what goes into running an AB test:
Let’s walk through each of these systematically.
Feature flagging is used to enable or disable a feature for a given user. And we can think of each AB test as a flag that determines which variant a user sees. That’s where randomization comes in.
Randomization is about “rolling the dice” and figuring out which variant the user sees. It sounds simple but it’s actually complicated to do well. Let’s start with the “naive” version of randomization to illustrate the complexity.
Naive version of randomization
A user hits the home page and we have a test we’re running on the main copy, with variants control and test. The backend has to determine which one to render to the user. Here’s how the randomization works for a user visiting the home page:
Math.random()
(or whatever randomization function exists for your language)Math.random()
. If it’s < 0.5, then assign the control variant. Otherwise assign the test variant.Simple enough. This is actually what we implemented at Storyblocks back in 2014 when we first started AB testing. It works but it has some noticeable downsides:
Improved randomization via hashing
So how do we do randomization better? The simple answer: hashing. Instead of simply rolling the dice using Math.random()
, we hash the combination of the experiment identifier and the user identifier using something like MD5, which effectively creates a consistent random number for the experiment/user combination. We then take the first few bytes and modulo by a relatively large number (say 10,000). Then divide your variants across these 10,000 “shards” to determine which variant to serve. (if you’re interested in actually seeing some code for this, you can check out Eppo’s SDK for it here). Here’s what that looks like in a diagram with 10 shards.
After you’ve computed the variant, you log the result, but instead of writing to a transactional database, which is blocking, you write the result to a data firehose (such as AWS Kinesis) in a non-blocking way. Eventually the data makes its way into a table in your data lake/warehouse for analysis (often called the “assignments” table).
Ok, so why do I need a feature flagging tool? Can’t I just implement this hashing logic myself? Yes, you could (and we did at Storyblocks back in the day) but there are some downsides
The answer to randomization: feature flagging
So what do we do? Feature flagging! I won’t go into it in full detail here, but feature flagging solves these issues for us, by combining the best of both worlds: the ability to opt specific groups of users into a test and the ability to randomize everyone else. There’s a great Eppo blogpost that describes what goes into building a global feature flagging service if you want to learn more.
Metrics are probably the easiest part of AB testing to understand. Each business or product typically comes with its own set of metrics that define user engagement, financial performance and anything else you can measure that will help drive business strategy and decisions. For Storyblocks, a stock media site, that was 30-day revenue for a new signup (financial), downloads (engagement), search speed (performance), net promoter score (customer satisfaction) and many more.
The naive approach here is simply to join your assignments table to other tables in your database to compute metric values for each of the users in your experiment. Here are some illustrative queries:
SELECT a.user_id, a.variant, SUM(p.revenue) AS revenue
FROM assignments a
JOIN purchases p
ON a.user_id = p.user_id
WHERE a.experiment_id = 'some-experiment'
AND p.purchased_at >= a.assigned_at
SELECT a.user_id, a.variant, COUNT(*) AS num_page_views
FROM assignments a
JOIN page_views p
ON a.user_id = p.user_id
WHERE a.experiment_id = 'some-experiment'
AND p.viewed_at >= a.assigned_at
-- etc.
This becomes cumbersome for a few reasons:
So to scale your AB testing, you need a system with the following:
Let me explain the event/fact layer in more detail. A critical aspect to making metrics easily reproducible and measurable is to base them on events or “facts” that occur in the product or business. These should be immutable and have a timestamp associated with them. At Storyblocks those facts included subscription payments, downloads, page views, searches and the like. The metric for 30-day revenue for a new signup is simply an operation (sum) on top of a fact (subscription payments). Number of searches is simply a count of the number of search events. And so on. A company like Eppo makes these facts and other definitions a core part of your AB testing infrastructure and also provides the capabilities for computing assignments once and building out a fact/metric repository.
An important aspect of configuring an experiment is defining primary and guardrail metrics. The primary metric for an experiment is the metric most closely associated with what you’re trying to test. So for the homepage refresh of YourDelivery where you’re testing blue vs red background colors, your primary metric is probably revenue. Guardrail metrics are things that you typically aren’t trying to change but you’re going to measure them to make sure you don’t negatively impact user experience. Stuff like time on site, page views, etc.
Ok, statistics. This is the hardest part for someone new to AB testing to understand. You’ve probably heard that we want a p-value to be less than 0.05 for a given metric difference to be statistically significant but you might not know much else. So I’m going to start with the naive approach that you can find in a statistics 101 textbook. Then I’ll show what’s wrong with the naive approach. Finally, I’ll explain the approach you should be taking. There will also be a bonus section at the end.
The naive approach: the Student t-test
Let’s assume we’re running the home page test for YourDelivery shown above, with two variants control (blue) and test (red) with an even 50/50 split between them. Let’s also assume we’re only looking at one metric, revenue. Every user that visits the home page will be assigned to one of the variants and then we can compute the revenue metric for each user. How do we determine if there’s a statistically significant difference between test and control? The naive approach is simply to use a Student t-test to check if there’s a statistical difference. You compute the mean and standard deviation for test and control, plug them into the t-statistic formula, compare that value to a critical value you look up, and voila, you know if your metric, in this case revenue, is statistically different between the groups.
Let’s dive into the details. The formula for the classic t-statistic is as follows:
Variable definitions in the formula are as follows:
To look up the critical value for a given significance level (typically 5%), you need to know the degrees of freedom. However for large sample sizes that we typically have when we’re AB testing, the t-distribution converges to the normal distribution so we can just use that to look up the critical value. The parameters for that normal distribution under the null hypothesis (i.e. there is no difference between the groups) are:
At Storyblocks this is the approach we used. Since we wanted to track how the test was performing over time, we would plot the lift and p-value over time and use that for making decisions.
What’s wrong with the naive approach
The naive approach seems sound, right? After all, it’s following textbook statistics. However there are a few major downsides:
The Peeking Problem
Using the naive t-test approach, we thought we were getting a 5% significance level. However, the classic t-test only provides the advertised statistical significance guarantees if you look at the results once (in other words, you pre-determine a fixed sample size). Evan Miller writes a great blog post about this problem that I highly recommend reading to understand more. Below is a table from Evan’s blog post illustrating how bad the peeking problem is.
So if you’re running a test for 2+ weeks and checking results daily, then to get a true 5% significance, you need to raise your significance to be ≤ 1%. That’s a pretty big change and represents at least a full standard deviation of difference from the naive approach.
The approach you should take
Ok, now that we know some pitfalls of the naive approach, let’s outline key aspects of the way we should approach the statistics for our AB testing (I’ll include more info about each below the list in separate sections).
(1) Relative lifts
The rationale behind relative lifts is straight forward: we typically care about relative changes instead absolute changes and they’re easier to discuss. It’s easier to understand a “5% increase in revenue” compared to a “$5 increase in revenue per user”.
How does the math change for relative lifts? I’m going to quote from Eppo’s documentation on the subject. First, let’s define relative lift:
From the central limit theorem, we know that the treatment and control means are normally distributed for large sample sizes. This allows us to model the relative lift as a normal distribution with the following parameters:
Ok, that’s somewhat complicated. But it’s necessary to compute the sequential confidence intervals.
(2) Sequential confidence intervals
First, let’s start with the confidence interval using a visual representation from an Eppo experiment dashboard:
So you can see that the “point estimate” is a 5.9% lift, with a confidence interval of ~2.5% on either side representing where the true relative lift should be 95% (one minus the typical significance of 5%) of the time. These are much easier for non-statisticians to interpret than p-values — the visuals really help illustrate the data and statistics together.
So what are sequential confidence intervals? Simply put, they’re confidence intervals that hold to a certain confidence level over all of time. They solve the “peeking problem” so you can look at your results as often as you want knowing that your significance level holds. The math here is super tricky so I’ll simply refer you to Eppo’s documentation on the subject if you’re interested in learning more.
(3) Controlled-Experiment Using Pre-Experiment Data (CUPED)
Sequential confidence intervals are wider than their fixed sample counterparts, so it’s harder for metrics to reach statistical significance when using sequential confidence intervals. Enter “Controlled-Experiment Using Pre-Experiment Data” (commonly called CUPED), a method for reducing variance by using pre-experiment data. In short, we can leverage what we know about user behavior before an experiment to help predict the relative lift more accurately. Visually, it looks something like the following:
The math is complicated so I won’t bore you with the details. Just know that powerful AB testing platforms like Eppo provide CUPED implementations out of the box.
Bonus material — simplifying computation
While I didn’t fully write out the math for sequential confidence intervals, know that we need to compute the number of users, the mean, and the standard deviation of each group, treatment and control, and we can plug those in to the various formulas.
First, the means are relatively simple to compute:
The standard deviation is slightly harder to compute but is defined as follows:
As you can see, we must first compute the mean and then go back and compute the standard deviation. That’s computationally expensive because it requires two passes. But there’s a reformulation we can employ to do the computation in one pass. Let me derive it for you:
Ok, that looks pretty complicated. The original formula seems simpler. However you’ll notice we can compute these sums in one pass. In SQL it’s something like:
SELECT count(*) as n
, sum(revenue) as revenue
, sum(revenue * revenue) as revenue_2
FROM user_metric_dataframe
So that’s great from a computation stand point.
Bonus material — Bayesian statistics
Perhaps you’ve heard of Bayes’ theorem before but you’ve likely not heard of Bayesian statistics. I certainly never heard about it until I arrived at Eppo. I won’t go into the details but will try to provide a brief overview.
Let’s start with Bayes’ theorem:
In Bayesian statistics, you have a belief about your population and then the observed data. Let’s simplify this to “belief” and “data” and write Bayes’ theorem slightly differently.
So basically you use the likelihood to update your prior, giving you the posterior probability (ignoring for a moment the normalization factor, which is generally hard to compute).
Why is this methodology potentially preferred if you have a small sample size? Because you can set your prior to be something that’s relatively informed and get tighter confidence intervals than you would with classical frequentist statistics. Referring back to the original example, you could say that you expect the relative difference between test (red) and control (blue) is normally distributed with standard deviation of 5% (or something like that, it’s a bit up to you to set your priors).
I totally understand that’s hard to follow if you have no knowledge of Bayesian statistics. If you want to learn more, I recommend picking up a copy of the book Bayesian Statistics the Fun Way. You could also read through the sections of Eppo’s documentation on Bayesian analysis and confidence intervals.
Drawing conclusions is the art of AB testing. Sometimes the decision is easy: diagnostics are all green, your experiment metrics moved in the expected direction and there were no negative impacts on guardrail metrics. However, studies show that only around 1/3 of experiments produce positive results. A lot of experiments might look similar to the report card below for a “New User Onboarding” test:
The primary metric “Total Upgrades to Paid Plan” is up ~6% while there are some negative impacts such as “Site creations” being down ~10%. So what do you do? Ultimately, there’s no right answer. It’s up to you and your team to make the tough calls in situations like this.
In addition to experiment report cards, it’s important to look at experiment diagnostics to make sure the underlying data is in good shape. A very common problem with AB testing is what’s called “sample ratio mismatch” or SRM, which is just a fancy way of saying that the number of users in test and control don’t match what’s expected. For instance you might be running a 50/50 test but your data is showing 55/45. Here’s what an SRM looks like in Eppo:
There’s also a variety of other ways your data could be off: one or more of your metrics may not have data; there could be an SRM for a particular dimension value; there may not be any assignments at all; there might be an imbalance of pre-experiment data across variants; and more.
Tools like Eppo help make your life easier by providing you easy-to-understand dashboards that are refreshed nightly. So you can grab your cup of coffee, open up your experiment dashboard, check on your experiments, and potentially start making decisions (or at least monitoring to make sure you haven’t broken something).
While you might have initially thought that building an AB testing platform is relatively straight forward, I hope I illustrated that doing it well is extremely challenging. Everything from building a feature flagging tool, to constructing a metrics repository, to getting the stats right, to actually computing the results on a nightly basis, there’s a lot that goes into a robust platform. Thankfully, you don’t need to build one from scratch. There are a variety of tools and platforms that help make AB testing easier.
Analyzing each of these platforms is beyond the scope of this article. Given all the requirements for an AB testing platform outlined above, however, I can confidently say that Eppo (even though I may be slightly biased because I work there) is the best all-in-one platform for companies that have their data centralized in a modern data warehouse (Snowflake, BigQuery, Redshift, or Databricks) and are looking to run product tests on web or mobile, including tests of ML/AI systems. Eppo provides a robust, global feature flagging system, a fact/metric repository management layer, advanced statistics, nightly computations of experiment results, detailed experiment diagnostics, and a user-friendly opinionated UX that is easy to use even for non-statisticians.
There’s a lot out there to read about AB testing. Here are some of my recommendations:
And that’s a wrap. Thanks for sticking around folks!